Re:Invent Amazon Web Services teased its next gen AI accelerator dubbed Trainium3 at re:Invent on Tuesday, which it says will deliver 4x higher performance than its predecessor when it arrives late next year.
Details on the part are still quite thin, however, speaking with The Register ahead of re:Invent, Gadi Hutt, director of product and customer engineering for AWS’ Annapurna Labs team, expects Trainium3 to be the first dedicated machine learning accelerator built on a 3nm process node and achieve a 40 percent improvement in efficiency compared to Trainium2, which a year after its own paper launch, is entering general availability — more on that in a bit.
In terms of performance, Amazon is vague about actual performance figures. Trainium3’s 4x performance improvement is based on a complete “UltraServer” configuration, which we’re told is still in development.
What we do know is that the Trainium2 UltraServer, which features 64 accelerators in total, delivers 83.2 petaFLOPs of dense FP8 performance. So in theory, a Trainium3 UltraServer should deliver 332.8 petaFLOPS of compute, though it isn’t clear at what precision.
We’ve reached back out to AWS for clarification, but if we had to guess, we’re probably looking at either 6-bit or 4-bit floating point math — something that Nvidia is bringing to market with Blackwell and AMD plans to introduce with the MI355X sometime next year.
Factor in sparsity, and Amazon’s next-gen UltraServers could potentially deliver more than 1.3 exaFLOPS of AI compute, assuming Trainium3 also supports the same 4x multiplier as its processor.
We’ve also been assured that these performance claims refer to peak compute performance — aka FLOPS — and not some nebulous AI benchmark. This is an important detail as depending on the AI workload, performance is dependent on a number of factors not just FLOPS. An increase in memory bandwidth, for instance, can result in large gains in large language model (LLM) inference performance, something we’ve previously seen with Nvidia’s bandwidth boosted H200 chips.
While Amazon is willing to tease performance and efficiency metrics, it has yet to share details on the chip’s memory load out.
If we had to guess, we’d get more detail on the part right around the time Amazon is ready to tease its next generation of AI ASICs.
Trainium2 readies for battle
While we wait for more details on Trainium3, Amazon is bringing its second generation of Trainium compute services to the general market.
Teased at re:Invent last year, Trainium2, which despite its name is actually both a training and inference chip, features 1.3 petaFLOPS of dense FP8 compute, 96 gigabytes of high-bandwidth memory capable of delivering 2.9 TBps of bandwidth apiece.
For reference, a single Nvidia H100 boasts just under 2 petaFLOPS of dense FP8 performance, 80GB of HBM, and 3.35 TBps of bandwidth.
The chip itself is composed of a pair of 5nm compute dies integrated using TSMC’s chip-on-wafer-on-substrate (CoWoS) packaging tech along with four 24GB HBM stacks.
Similar to Google’s Tensor Processing Units (TPUs), these accelerators are bundled up into rack-scale clusters. Sixty-four Trainium2 parts spread across two inter-connected racks.
As we mentioned earlier, this Trn2 UltraServer configuration is capable of churning out 83.2 petaFLOPS of dense FP8 performance or 332.8 petaFLOPS with its 4x sparsity mode enabled.
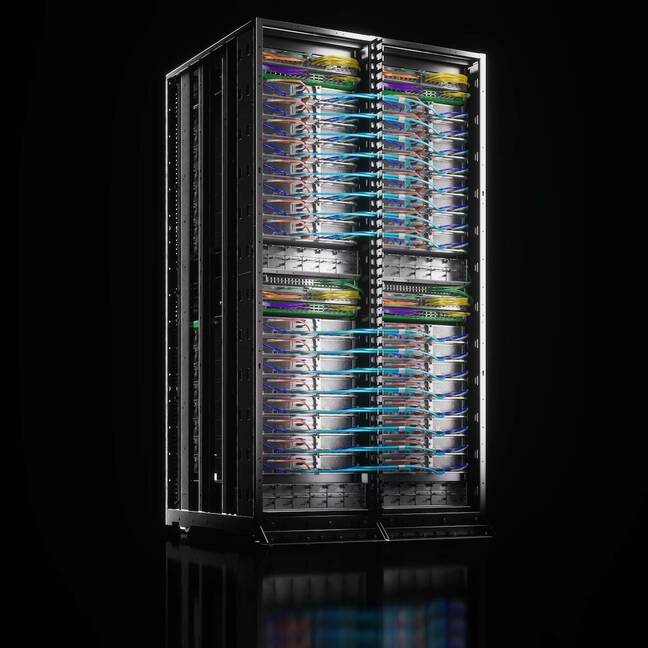
Here’s a closer look at AWS’ new Trn2 UltraServers, which boast 64 Tranium2 chips across two racks. – Click to enlarge
If that’s more compute than you’re looking for, Amazon also offers a Trn2 instance with 16 accelerators and about 20.8 petaFLOPS of dense compute.
According to Amazon, these instances offer 30 to 40 percent better price-performance over the current generation of GPU-based instances available on EC2 — specifically its Nvidia H200-based P5e and P5en-based instances.
For those using the chips to train models, Trainium2 can scale to even larger clusters with 100,000 or more chips. This is exactly what AWS and model builder Anthropic plan to do under Project Rainier, which will involve “hundreds of thousands” of Trainium2 chips producing “5x the number of exaFLOPS used to train their latest generation of AI models.”
Trn2 instances are now available in AWS’ US East (Ohio) with availability in additional regions coming in the near future. Meanwhile the larger Trn2 UltraServer config is currently available in preview.
Hedging their bets
While AWS’ Annapurna Labs team pushes ahead with custom silicon, it isn’t putting all of its eggs in one basket. The cloud giant already supports a wide variety of instances including H200, L40S, and L4 accelerators, and it is in the process of deploying a massive cluster of Blackwell parts under Project Ceiba.
Based on Nvidia’s Grace-Blackwell Superchips (GB200), the massive AI supercomputer will boast some 20,736 Blackwell GPUs, each connected by an 800 Gbps (1.6 Tbps per Superchip) of Elastic Fabric Adapter bandwidth.
In total, the machine is expected to produce roughly 414 exaFLOPS of super low precision sparse FP4 compute. However, we’ll note that precision will almost exclusively be used for inferencing, with higher precision FP8 and FP/BF used for training. For training, we expect Ceiba will still deliver a whopping 51 exaFLOPS dense BF16 compute or twice that if you’re willing to drop down to FP8.
In any case, while AWS may be pushing ahead with its Trainium silicon, it’s by no means done with Nvidia just yet. ®