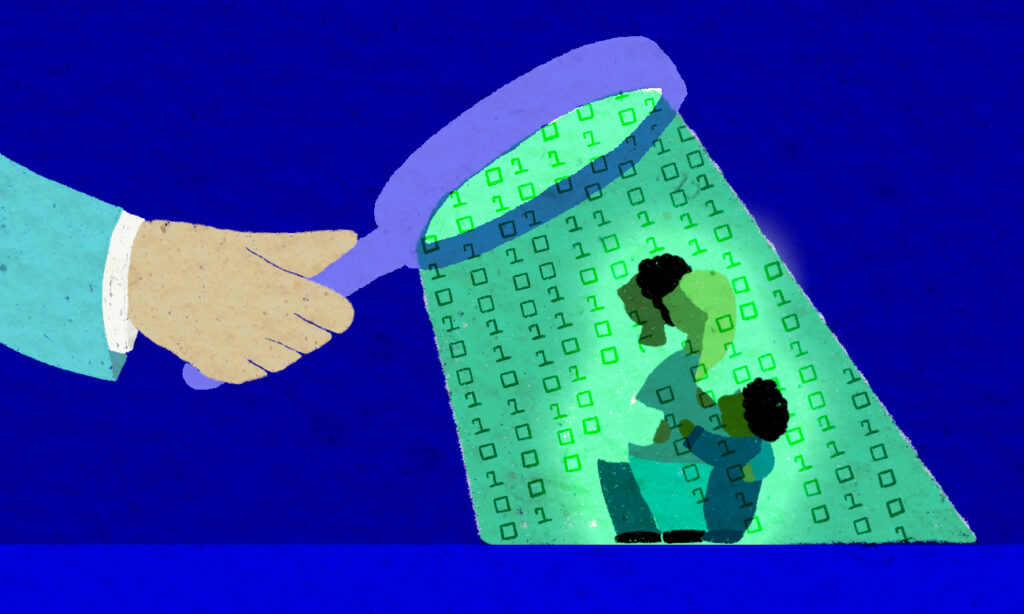
A study recently published by a University of Georgia professor unpacks how artificial intelligence tools are being deployed in the child welfare system, and examines the effectiveness of the approach.
The four-year study looked at how advanced algorithms are being used to assess risk in child welfare investigations, a method that has been controversial in some professional circles.
Author Daniel Gibbs — a former children’s attorney and an assistant professor at the university’s School of Social Work in Athens — is a specialist in the data behind how choices are made in the foster care and mental health care systems. His study, published in the Journal of Technology in Human Services in September, examined the use of an artificial intelligence tool to screen reports of child maltreatment in two urban counties in a western state. The precise location was kept confidential by the study and its author.
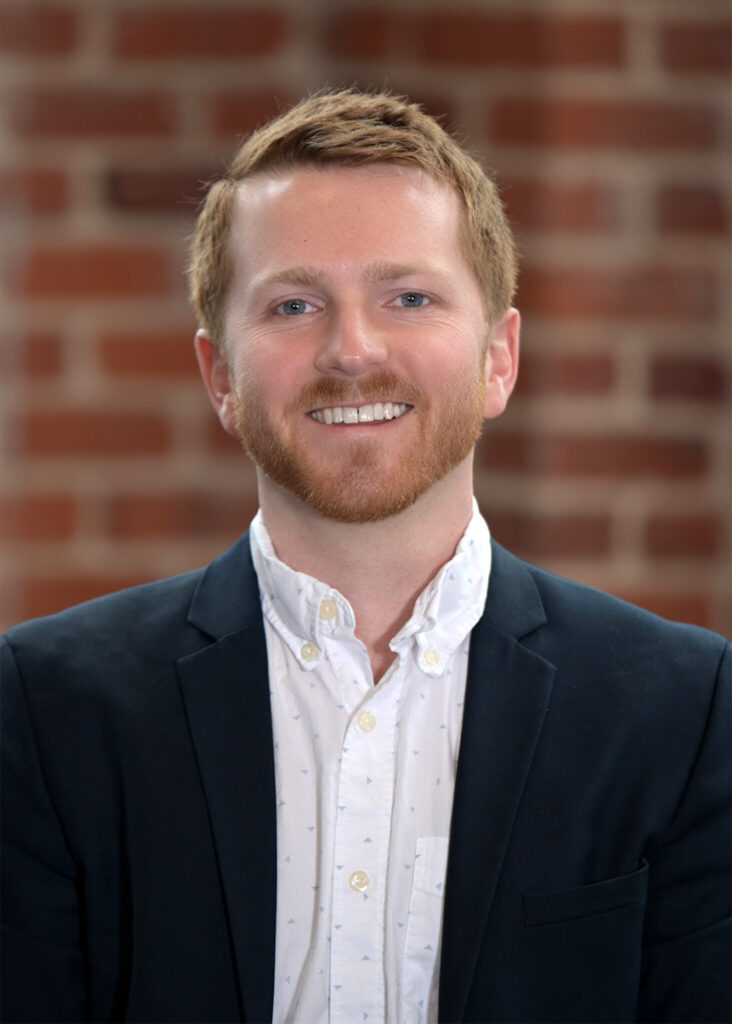
The “decision-making” tool examined in the paper predicts the risk that a child will be placed in foster care within two years, and provides county workers a risk score between 1 and 20. The higher the score, the higher the child’s risk of being abused or neglected.
Although the specific tool was not identified, it’s similar to the Allegheny Family Screening Tool that has been deployed in Pennsylvania since 2016. Child welfare algorithms such as these cull data from public benefit programs, criminal, medical, mental health, welfare and education records.
Proponents claim the method has helped social workers decide which children need greater protection in foster homes and court supervision, and who can be left safely at home with parents. But critics say its reliance on massive databases and population-level analyses of individuals’ lives leads to racial discrimination and too many kids pulled from their families.
Although Gibbs said he was interested in critiques of decision-making tools, the study examined how the tools are used, and whether study participants find them useful. But in an interview with The Imprint he acknowledged that the data feeding the algorithm “only reflects people who are known to these public systems,” and “may not reflect the full spectrum of experience.”
“I’ve not been convinced, personally, that we can overcome some of the historical bias that this data reflects,” Gibbs said. “But I also don’t think it’s a dead end. I just think that we’re going to have to get really creative with how we get better and better data to feed into these systems.”
Below is more from that conversation, which has been lightly edited for clarity.
For those who haven’t read your recently published paper, what are the main takeaways you’d want them to understand?
The biggest takeaway is there’s disagreement about the pros and cons of using AI for decision-making. On one hand, humans are incredibly inconsistent in our decision-making and the folks that bear the consequences of those unreliable, inconsistent decisions are kids and families.
Either we miss the needle in the haystack and a child or family experiences some sort of harm, or we grab too much hay and families get this intrusive intervention. On the other hand, there are real concerns about whether our data’s good enough to do this and what biases may be reinforced when we use AI.
Regardless of where we land on that, the impact of using AI in child welfare is always going to be mediated by how it’s actually used by human beings in the system. You can make a perfect tool with no error, no bias — and then practitioners don’t trust it, or they figure out ways around it. You also have the flip side of that, which is that our workforce may also mediate the outcomes of a bad tool.
It’s not simply about math and creating the most powerful AI model possible. It’s about how it gets used in practice. That’ll really determine what its impacts are.
“Either we miss the needle in the haystack and a child or family experiences some sort of harm, Or we grab too much hay and families get this intrusive intervention.”
— Daniel Gibbs, University of Georgia
For the general public, can you please lay out exactly what the current decision-making tools are that you discuss in your paper? How are they used in CPS intake and assessments?
So in these two counties, it was not an automatic algorithm decision. And what I mean by that is the algorithm was gathering public records and then weighing them to provide a risk score, but the algorithm was not making the screening decision. What it was doing was just producing a number between 1 and 20, and that was being used basically as another piece of information by the teams in this case that were making the screening decision — so really a supplement to human judgment, rather than a replacement for human judgment.
In this era of AI, are algorithmic tools at the front-end of the system inevitable?
I do think AI is really transforming so many parts of life. I think a couple years ago, I would have said maybe the AI was a thing we tried and kind of lost enthusiasm for.
But I think our human decision-making is always going to be limited, and so we’ll always be turning to things like this. I think it is inevitable that we use more tech, more data, more AI, and honestly, it should be.
There’s a lot of debate about AI and things like this, but you’d be hard-pressed to find people that think the status quo is great in terms of our decision-making for families. Knowing that, I think we’re only going to see more integration of this kind of technology.
What are some of the barriers that prevent child welfare workers from adopting or using decision-making tools?
The tool itself has to be useful. It has to offer some sort of advantage over the current way of doing things. If it doesn’t add some usefulness to the people doing it or using it, eventually you stop wasting your time, right?
It also really has to fit the context, so basically, the way that a tool matches with what you have to do. Even if it’s really good at predicting an outcome, for example, in child welfare, you have to have reasons for the decisions you make. There are high stakes in them. And so what that means, in terms of algorithms, it needs to be somehow explainable and follows some logic. If it doesn’t have those features, I think it will always be limited in how folks will adopt it.
Also, a lot of workers didn’t really understand how the tool was coming up with what it was coming up with, and it really undermined their trust. Particularly when it was a middle score, they were like, ‘Even if I did trust it, I wouldn’t know what to do with that.’
“It’s not simply about math and creating the most powerful AI model possible. It’s about how it gets used in practice. That’ll really determine what its impacts are.”
— Daniel Gibbs, University of Georgia
So sometimes it’s only really useful in the extremes. A 1 out of 20, if you saw other concerning factors, was hard to listen to. Because you can’t say, this infant has unexplained fractures but it’s a 1 of 20, so we should probably screen this out, right?
Whereas, if you say, this family, we don’t really have any big concerns, but it did come back at 20 out of 20. Maybe we should give it a second look.
Groups such as the ACLU have criticized use of big-data tools in the child welfare system that could further racial discrimination and marginalization of people who live in impoverished and over-policed communities. How do you view this issue?
Data isn’t biased. But the way that we enter it, and the practice that it reflects, certainly can be. And we have pretty good evidence that it is likely in a lot of places. One of the ways that we have to address this is really extensive testing before we deploy an algorithm.
One of the things is making sure that any sort of patterns in the data that might be problematic, that we’re transparent, that we’re testing these things.
So not just predictive accuracy, predicting the right thing. Is it equally good at sifting through cases to find children at risk as it is filtering out families that are not at risk? Or white families or Black families or single-parent families —all these things that if it wasn’t equally predictive across those groups, you might have a problem.
Last year, the Biden Administration released an Executive Order directing agencies to combat algorithmic discrimination. It built on a 2022 White House paper on AI that raised concern about the transparency of these tools in the nation’s child protection system. For tools like the ones you’ve studied, what is owed to the parents being screened, and their communities?
I think this is a really, really important question. If you asked the majority of parents, would they be comfortable being more or less surveilled by an algorithm for risk of harming their children, probably nine out of 10 of them would say no. So I think that creates a potential justice issue.
Child welfare is tricky because we serve families, but we protect children. That’s a complicated, ethical problem. Because at the end of the day, I think there are folks who would say the one person who benefits or loses the most from our bad decisions can’t consent to algorithms, which is a child. So that’s hard.
However, the folks with the constitutional right to raise their children however they want to, within limits, are parents, and we are using an algorithm to determine the direction of something that may infringe on that fundamental constitutional right. We have to engage with families and communities from the very beginning — before we ever start plugging their information into an algorithm — and getting their voices about what sort of algorithms they would be comfortable with, what data they would be comfortable with us using.
I think at a minimum, parents need to know that an algorithm was used in a decision in their case. I think some sort of notice is really important, but it’s not a meaningful notice unless you’re also equipping the frontline professionals in the system to explain to them what it means.
Now that you’ve completed this study, what is your opinion on the use of AI in child welfare?
I think AI is extraordinarily powerful in its ability to improve or really decrease the quality of our decision making. So with that, I think we have to be extraordinarily careful and creative in how we deploy it, using a ton of evaluation not just about its accuracy, but about its equity, about its transparency. We also should not be so concerned about those things that we fail to adopt innovations that are needed. I don’t think there’s any world in which we can’t capitalize on its potential benefits. It would be very unwise of us not to explore that carefully — emphasis on carefully.