Computer scientists claim they’ve come up with a way to thwart machine learning systems’ attempts to digitally manipulate images and create deepfakes.
These days you can not only create images from scratch using AI models – just give them a written description, and they’ll churn out corresponding pics – you can also manipulate existing images using the same technology. You can give these trained systems a picture and describe how you want it altered, or use a GUI to direct them, and off they go.
That creates the potential for a terrifying future in which social media is flooded with automated mass disinformation, or people are blackmailed over snaps twisted by machines. If no-one can tell the fakes from the real thing, they can be just as damaging.
One approach to prevent that is to watermark or digitally sign images and other content so that manipulations are detectable. Another path – proposed by the brains at MIT – is to engineer snaps so that AI models can’t alter them at all in the first place.
“Consider the possibility of fraudulent propagation of fake catastrophic events, like an explosion at a significant landmark,” said Hadi Salman, a graduate student in the university’s department of electrical engineering and computer science who worked on this project.
“This deception can manipulate market trends and public sentiment, but the risks are not limited to the public sphere. Personal images can be inappropriately altered and used for blackmail, resulting in significant financial implications when executed on a large scale.”
Salman may well be right. Deepfakes have already been used to spread misinformation, or trick and harass netizens, and AI-crafted images have become increasingly realistic. As the technology improves in the future, people will be able to create videos with realistic false audio too, he warned.
That’s why Salman and his colleagues decided to develop machine learning software, dubbed PhotoGuard, to prevent miscreants from misusing these tools to manipulate content.
Images are basically processed by ML models as arrays of numbers. PhotoGuard tweaks these arrays in order to hamper other machine learning models from correctly perceiving the input image – so that ultimately the picture cannot be manipulated. The pixels are left untouched as far as people are concerned, so the protected image looks the same to the human eye – but not to a computer.
In the example shown below, a photo shows US TV funnymen Trevor Noah and Michael Kosta at the US Open. The original – unprotected – photo can be manipulated by a text-driven diffusion model to make them appear in a completely different setting: in a ballroom dancing together. However, with the PhotoGuard alterations applied, the diffusion model is thrown off by the invisible perturbations, and can’t fully alter the image.
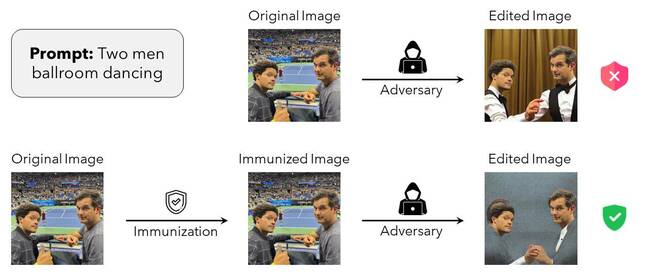
Figure from the MIT PhotoGuard paper outlining how this immunization of data from manipulation basically works – Click to enlarge
“In this attack, we perturb the input image so that the final image generated by the [large diffusion model] is a specific target image (eg, random noise or gray image),” the academics wrote in their non-peer-reviewed paper describing PhotoGuard, released on ArXiv.
Strictly speaking, that approach is referred to as a diffusion attack by the MIT team. Its goal is to bamboozle the image-manipulating AI and make it effectively ignore whatever text prompt it was given.
There is a second, simpler technique called an encoder attack, which tries to make the manipulating AI perceive a photo as something else – such as a gray square – which thwarts its automated editing. The result is more or less the same, either way: attempts to use AI to manipulate the images go off the rails.
“When applying the encoder attack, our goal is to map the representation of the original image to the representation of a target image (gray image),” the team wrote in their paper. “Our (more complex) diffusion attack, on the other hand, aims to break the diffusion process by manipulating the whole process to generate an image that resembles a given target image (gray image).”
They carried out their experiments against Stability AI’s Stable Diffusion Model v1.5, and noted that although PhotoGuard can protect images from being digitally altered by AI, it’s difficult to apply practically. For one thing, the diffusion attack requires considerable GPU capacity – so if you don’t have that kind of silicon to hand, you’re out of luck.
Really, the responsibility should lie with the organizations developing these AI tools rather than individuals trying to safeguard their images, the researchers assert.
“A collaborative approach involving model developers, social media platforms, and policymakers presents a robust defense against unauthorized image manipulation. Working on this pressing issue is of paramount importance today,” argued Salman.
“And while I am glad to contribute towards this solution, much work is needed to make this protection practical. Companies that develop these models need to invest in engineering robust immunizations against the possible threats posed by these AI tools. As we tread into this new era of generative models, let’s strive for potential and protection in equal measures.”
PhotoGuard isn’t a perfect solution. If an image is available for anyone to download without any protections applied to it, a miscreant could grab that unprotected version of the photo, edit it using AI, and distribute it. Also, cropping, rotating, or applying some filters could break PhotoGuard’s perturbations.
The MIT bods said they are working to try and make their open source software (MIT licensed, natch) more robust against such modifications.
The Register has asked Salman for further comment. ®