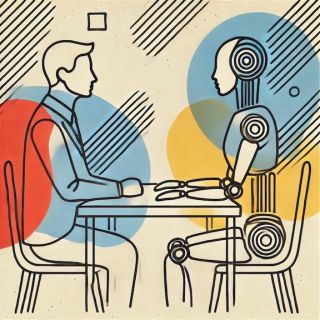
Source: Art: DALL-E / OpenAI
Just who (or what) wrote what? This seems to be a prevailing and resonant question that seems to be focused on author over content.
Recent research has uncovered a significant challenge in this curious authorship debate of artificial intelligence (AI): neither humans nor AI systems are consistently able to detect AI-generated content in online conversations. This finding might have significant implications for digital communication, online trust, and the future of human-AI interaction. Or, on the other hand, it might just be a wild goose chase that is intended to drive a wedge between human and AI creativity and productivity.
A New Take on the Turing Test
Researchers conducted a novel experiment, expanding on the classic Turing test. They presented transcripts of conversations between humans and AI to both human participants and AI models, asking them to determine which was which. The results were striking and unexpected.
Displaced human judges (those reading transcripts) performed no better than chance in identifying AI participants. Even more surprisingly, AI models like GPT-3.5 and GPT-4, when tasked with the same identification, showed similarly poor performance. Perhaps most intriguingly, the most advanced AI conversationalist was more likely to be judged as human than actual human participants.
Blurring Lines in the Digital Landscape
These findings suggest that as AI language models become more sophisticated, the line between human- and AI-generated content is becoming increasingly blurred. This has important implications for our digital interactions and raises important questions about the nature of online communication.
As AI systems become more prevalent in digital spaces, it may become increasingly difficult to discern whether we’re interacting with humans or machines. This challenge extends beyond mere curiosity—it can strike at the heart of digital trust. How can we verify the source of information or the identity of those we’re communicating with in an environment where AI can convincingly mimic human discourse?
The Hunt for Reliable Detection Methods
The study also explored various methods of AI detection, including statistical approaches and the use of AI to detect other AI. While some methods showed promise, they all had significant limitations.
- Statistical Approaches: Statistical methods could identify some patterns in AI-generated text but struggled with more advanced models. As AI language models improve, these statistical signatures become increasingly subtle and difficult to detect reliably.
- AI Detecting AI: AI detectors performed better than chance but still made many errors, especially with more sophisticated AI-generated content. This suggests that even AI, trained specifically for this task, struggles to consistently identify its own kind in conversational settings.
- The Human Element: Interestingly, interactive human interrogators performed better than those reading transcripts, but they still struggled to consistently identify AI participants. This highlights the value of direct interaction in detecting AI but also underscores the sophistication of modern AI language models.
Does It Really Matter?
As we grapple with the challenges of AI detection, a question emerges: Does it really matter? In an increasingly AI-integrated world, the distinction between human and AI-generated content might become less relevant in many contexts. Consider how we’ve seamlessly integrated spell-check and autocorrect into our writing process—we rarely pause to consider whether a correctly spelled word is the result of human knowledge or technological assistance. Similarly, as AI becomes more deeply woven into our digital interactions, we might find ourselves focusing less on the origin of the content and more on its value and relevance.
This perspective doesn’t negate the importance of transparency in high-stakes situations, but it does suggest that in many everyday interactions, the pursuit of distinguishing between human and AI contributions might be unnecessary or even counterproductive. Instead of “chasing the author,” we might be better served by developing frameworks to evaluate the quality, ethics, and impact of digital content, regardless of its origin. This shift in focus could lead to more productive discussions about how we can harness the combined potential of human and artificial intelligence to enhance our digital experiences and decision-making processes.
Embracing Complexity
The difficulty in distinguishing between human and AI communication underscores the remarkable progress in AI technology. However, it also highlights the complex challenges we face in an increasingly AI-integrated world. Today, and into the future, it will be crucial to approach these challenges with nuance, balancing the potential benefits of AI with the need for transparency, trust, and human-centered design in our digital ecosystems.
In the end, this research doesn’t just reveal our limitations in detecting AI—it opens up new questions about the nature of communication, intelligence, and what it means to be human in a world where machines can convincingly mimic our most distinctive trait: the ability to converse.